Tyler Von Harz, Community Partner
Feb 6, 2025
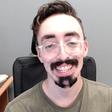
Spreadsheets have been a staple in business operations for decades, but they weren’t built for the scale, speed, and complexity of modern data analytics. Organizations relying on them for critical decision-making often struggle with slow, error-prone processes, fragmented data, and an inability to gain real-time insights.
Instead of enabling smarter decision-making, outdated data systems create roadblocks that limit growth and efficiency.
Data modernization is the process of transforming and optimizing your organization's data systems, architecture, and practices to meet contemporary demands for agility, scalability, and advanced analytics.
So, what does that even mean?
Upgrading legacy infrastructures to more modern, cloud-based platforms, enabling efficient data management and real-time processing. Doing this properly means you can suddenly harness the full potential of your data assets, and with that comes better decision-making and operational efficiency.
Why does data analytics modernization matter?
Businesses are increasingly moving away from outdated legacy systems to embrace modern data analytics platforms. Driven by the need for real-time insights, improved scalability, and the ability to leverage advanced analytics and artificial intelligence, they are embracing data analytics modernization to gain a massive competitive edge.
Modernizing data analytics lets businesses process and analyze data quicker, encouraging more timely and informed decisions. Having such agility also enhances operational efficiency, allowing organizations to optimize processes and reduce costs. Plus, modern analytics platforms support advanced capabilities such as predictive analytics and machine learning, further driving innovation and strategic advantage.
Clinging to legacy systems can create significant bottlenecks and limit an organization's ability to innovate. Outdated infrastructures often struggle with data silos, slow processing speeds, and limited integration capabilities, all of which slow the flow of information. Missed opportunities and an inability to meet evolving customer expectations that come about as a result, will ultimately affect the company’s competitiveness in the market.
Data architecture modernization: how the new compares to the old
The evolution from traditional to modern data architectures marks a significant shift in how organizations manage, process, and utilize data.
Traditional vs. modern data architectures
Traditional data architectures are typically characterized by monolithic, on-premise data warehouses. Often operating in silos with tightly coupled storage and compute resources, these environments can be slow, and scaling up often requires substantial investment in hardware and infrastructure. Additionally, integrating diverse data sources can be cumbersome, limiting the ability to perform comprehensive analytics.
In contrast, modern data architectures embrace highly distributed, cloud-native environments. They leverage cloud computing to provide scalable storage and compute resources, so organizations can handle vast amounts of structured and unstructured data efficiently.
This approach also supports real-time data processing and advanced analytics. The decoupling of storage and compute resources allows for independent scaling, optimizing performance and cost efficiency.
The adoption of microservices, containerization, and serverless technologies further enhances modern data architectures. Microservices break down applications into smaller, independent services that can be developed, deployed, and scaled individually, promoting flexibility and resilience.
Containerization, through platforms like Docker and Kubernetes, provides consistent environments for deploying applications, ensuring reliability across different stages of development and production. Serverless computing abstracts infrastructure management, allowing developers to focus on code execution without worrying about underlying servers, leading to increased agility and reduced operational overhead.
What is the need for a modern data stack?
“Information is the oil of the 21st century, and analytics is the combustion engine.” - Peter Sondergard.
A modern data stack is a collection of tools and technologies designed to facilitate the efficient ingestion, storage, processing, and, most importantly, the final analysis of data. Each layer of this stack plays a crucial role:
- Ingestion – the tools that collect and import or “ingest” your data from various sources into the system.
- Storage – scalable repositories that securely store large volumes of data.
- Processing – frameworks that transform raw data into structured formats suitable for analysis.
- Analytics – platforms that allow you to explore your data, create visualizations, and perform advanced analytics.
Implementing a modern data stack is essential for organizations aiming to remain competitive and make data-driven decisions. It provides the scalability to handle growing data volumes, the flexibility to adapt to changing business needs, and robust support for machine learning initiatives.
Why do basic spreadsheets fail in modern data stacks?
Many organizations resist modernizing because spreadsheets appear to be familiar, functional, and "free." Employees are comfortable with them, and IT teams may not want to overhaul established workflows. However, as data volumes grow and complexity increases, spreadsheets become a liability rather than an asset.
One of the biggest challenges with spreadsheets is their inability to scale. As data volumes grow, spreadsheets become increasingly sluggish, often crashing or slowing down when handling large datasets. Unlike modern cloud-based platforms that separate storage and computing for optimal performance, spreadsheets force everything into a single file, making them inefficient for real-time data analysis. They also require manual updates, increasing the risk of human error. With no built-in automation, employees must spend hours copying and pasting data, double-checking formulas, and manually reconciling inconsistencies—time that could be spent on higher-value analysis.
Another major issue is how spreadsheets trap data in silos. Since they operate as static files rather than connected systems, they prevent seamless integration across an organization. Data is often stored in multiple versions across different departments, leading to inconsistencies and conflicting reports.
Traditional spreadsheets also lack real-time synchronization with other data sources, meaning their insights are often outdated before they’re even analyzed. This creates a fundamental problem: decision-makers are basing their strategies on fragmented and stale data rather than a unified and continuously updated view of the business.
Yet many companies still cling to their legacy spreadsheets.
Despite these challenges, many organizations stick with spreadsheets due to habit, perceived cost savings, or fear of migrating to a new system. But what seems like a cost-effective solution often leads to hidden inefficiencies, wasted productivity, and inaccurate reporting that can have serious financial consequences.
Modernizing data analytics means moving away from static, error-prone spreadsheets and adopting cloud-based platforms that enable automation, scalability, and real-time collaboration. Businesses that continue to rely on legacy spreadsheets will find themselves falling behind competitors that leverage AI, machine learning, and advanced analytics for faster, more reliable decision-making.
Developing a data modernization strategy
Developing a comprehensive data modernization strategy is essential for organizations aiming to enhance their data infrastructure and analytics capabilities. A well-structured strategy encompasses clear objectives, stakeholder alignment, a realistic timeline, and measurable success metrics.
Consider the essentials
A robust data modernization strategy begins with defining specific objectives, such as improving data accessibility, enhancing analytics capabilities, or reducing operational costs. Engaging key stakeholders from various departments ensures the strategy aligns with organizational needs and secures necessary support.
Developing a realistic timeline with achievable milestones is crucial, considering factors like resource availability and system complexity. Establishing quantifiable success metrics, such as performance improvements or cost savings, allows for effective progress assessment.
When planning the migration, organizations often choose between a "big bang" approach and a phased migration. The big bang approach involves transitioning all systems simultaneously, which can be quicker but carries higher risks due to the immediate changeover. In contrast, a phased migration allows for gradual implementation, reducing risk by enabling testing and adjustments at each stage.
Governance, security, and compliance
Implementing robust data governance frameworks is vital for maintaining data quality, security, and compliance with regulations such as the General Data Protection Regulation (GDPR) and the California Consumer Privacy Act (CCPA). Key components include data catalogs for organized data management, lineage tracking to monitor data flow, and adherence to privacy regulations to protect personal information.
Modern data automation platforms enhance security through features like encryption, role-based access controls, and real-time threat detection, ensuring that data is both accessible to authorized users and protected against unauthorized access.
Data modernization services
To accelerate the modernization process and mitigate risks, organizations can leverage professional or managed services. Consulting services can provide guidance to assess current data environments and develop tailored strategies.
Cloud migration services assist in transitioning data and applications to cloud platforms, ensuring scalability and flexibility. Data warehouse re-platforming involves upgrading or replacing legacy data warehouses with modern solutions to improve performance and analytics capabilities. Engaging in these services can help you stick to best practices.
Many times, picking the right tools is the best way to make things easy for yourself. Transitioning from traditional spreadsheets to advanced tools like Quadratic can enhance data management by offering more robust features and better integration capabilities, supporting the overall data and analytics modernization effort.
Final thoughts
Data analytics modernization is essential for businesses that want to stay competitive in a world where real-time insights drive smarter decisions. Moving beyond outdated legacy systems, including error-prone spreadsheets, allows organizations to harness the full power of modern data architectures, scalable platforms, and AI-driven analytics.
For organizations still relying on traditional spreadsheets, transitioning to more intelligent solutions like Quadratic can be a practical first step toward analytics modernization. Unlike conventional spreadsheets, Quadratic offers better integration, automation, and scalability—bridging the gap between familiarity and innovation.