MJ Lindeman, PhD, Community Partner
Mar 26, 2025
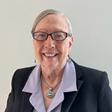
In the world of data analysis, the saying "garbage in, garbage out" remains painfully true. Before any meaningful analysis can begin, data must be clean, consistent, and properly formatted. Data scientists and business intelligence professionals acknowledge that the quality of your data limits the data stories and actionable insights you can get from datasets.
Three terms are not clearly distinguished: data preparation, data wrangling, and data cleaning. Data cleaning is the process of identifying and correcting errors, inconsistencies, and inaccuracies in datasets. It is traditionally one of the most time-consuming aspects of analytics.
Data preparation is a broader term that includes converting data between formats, combining data from different sources, and other changes necessary to create a proper dataset for analysis. In practice, data wrangling tends to emphasize the more creative, exploratory aspects of working with difficult datasets.
The importance of data cleaning is often unrecognized within an organization. The impact of poor-quality data extends far beyond being an inconvenience. A Gartner study found that poor data quality costs organizations an average of $12.9 million annually. Effective cleaning data methods are essential business investments rather than optional technical exercises.
This blog post discusses data cleaning tools and techniques, from traditional solutions to modern AI-powered approaches, and explains how newer platforms, such as Quadratic, are revolutionizing the way we handle messy data. Much of the manual labor previously needed can now be eliminated entirely.
The data cleaning challenge
Raw data is rarely analysis-ready. Common issues include:
- Missing values: Empty cells or null values create gaps in analysis that can skew analytical results and lead to decisions with negative consequences.
- Duplicate records: Redundant entries can skew calculations and waste resources by impacting aggregate measures such as calculations of totals and averages.
- Inconsistent formatting: Varying date formats, numerical notations, or text capitalization need to be standardized when in the same dataset.
- Outliers and errors: Values outside the expected range or containing obvious mistakes may either be valid observations or errors that require context and domain knowledge to recognize.
- Structural problems: Merged cells, improper headers, or inconsistent table structures can cause major problems when grouping or filtering data.
There is a very real business impact of data cleaning challenges. Consider how many issues and problems could come from this example data for just three entries with four fields: Name, Email, Purchase_Date, and Amount.
NAME | PURCHASE_DATE | AMOUNT | |
---|---|---|---|
John Smith | john.smith@mail.com | 01/05/22 | $1,200 |
John smith | johnsmith@email.com | 1/5/2022 | 1200 |
J. Smith | john.smith@mail.com | Jan 5 2022 | $1,200.00 |
After data cleaning, the three lines become one corrected entry.
NAME | PURCHASE_DATE | AMOUNT | |
---|---|---|---|
John Smith | john.smith@mail.com | 2022-01-05 | $1,200.00 |
This simple example demonstrates how messy data can create artificially inflated customer counts, inaccurate sales reporting, and missed opportunities for personalization. The cleaning process (1) unified multiple representations of the same customer, (2) standardized email and date formats, and (3) normalized monetary values.
These transformations are essential for accurate analytics. If you need to make a business case for improving data quality, Gartner defines the five steps of creating a business case. McKinsey provides a best-practice data governance model to help organizations create business value. They note, "Data processing and cleanup can consume more than half of an analytics team’s time, including that of highly paid data scientists, which limits scalability and frustrates employees."
Data cleaning tools
There is a strong move to use automation to prepare data for analysis. This includes (1) cleaning data, such as eliminating errors and inconsistencies, and (2) preprocessing, which includes transformation, integration, and enrichment. These can be combined into a data pipeline that moves data from a source to a destination.
There are two types of pipelines for moving data through this process: (1) the traditional ETL (Extract, Transform, Load) pipeline and (2) the newer ELT (Extract, Load, Transform) pipeline. Both ETL and ELT have advantages and disadvantages. Which is better to use depends on the details of the process being automated.
Numerous available tools assist in cleaning and preparing data for analysis, and some are designed for particular types of data in specific industries. For example, Enlitic's ENDEX standardizes medical image data for a radiology department.
More general tools handle data that is structured (such as tables) and unstructured (such as email) in a variety of formats. Organizations should evaluate tools based on their capabilities, ease of use, scalability, integration options, and cost.
There are many ways to identify and evaluate which tools are appropriate for your organization and specific needs. For example, the list of data quality tools on g2.com has comprehensive information regarding software solutions designed to enhance the accuracy, reliability, and consistency of your data.
It describes almost 200 software products that offer diverse functionalities, from data cleansing and matching to validation and monitoring. Each product profile provides detailed insights, including user reviews, scores, and comparisons, to help you identify the best fit if you have niche requirements.
Some popular paid tools include Excel and Alteryx Designer Cloud (formerly Trifacta). Open-source tools include OpenRefine and Python (Pandas). Quadratic AI is a modern, free solution that combines the power of built-in Python with native AI to quickly identify patterns in your data and clean it in seconds from a simple natural language query.
Comparison of data cleaning tools
1. Quadratic: The future of data analysis
Quadratic represents a paradigm shift in how we approach data cleaning. Unlike traditional tools that require extensive manual data cleaning methods, Quadratic's AI-powered platform fundamentally changes the equation. Its innovative approach means data cleaning is not necessary in the traditional sense when analyzing data in Quadratic.
There are several reasons why Quadratic stands apart from traditional data cleaning tools:
- AI-powered data recognition: Automatically identifies patterns in dirty data, eliminating manual cleansing
- Smart schema enforcement: Ensures data conforms to expected patterns
- Python integration within spreadsheets: Combines the accessibility of spreadsheets with powerful programming capabilities
- Version control: A coming-soon feature that tracks changes to data, making it easy to revert unwanted modifications
For organizations seeking to eliminate the data-cleaning bottleneck entirely, Quadratic offers a compelling solution that merges AI data-cleaning capabilities with intuitive spreadsheet functionality.
2. Excel: The familiar workhorse
Microsoft Excel remains the most widely used tool for data cleaning in data analytics. Its accessibility and familiar interface make it a go-to solution for many analysts. While not as automated as specialized data cleansing software, Excel's ubiquity and low learning curve make it suitable for smaller datasets and teams without specialized data skills. The following table compares Quadratic with Excel and its external PowerQuery.
Feature Description | Quadratic | Excel PowerQuery (External) |
---|---|---|
Native AI Integration | Built-in AI capabilities that automatically recognize, validate, and correct data without manual intervention | Copilot integration (still maturing) |
Seamless Python Integration | Write Python directly in spreadsheet cells alongside your data, combining programming power with spreadsheet accessibility | Write in Microsoft’s proprietary M script when coding changes to existing transformations or when defining a new transformation |
Access point | Browser-based | Desktop |
Version control | Built-in version control similar to software development tools, making it easier to track changes, revert modifications, and understand data lineage | Excel PowerQuery does not have version control |
Data visualizations | Built-in Plotly library to create or generate charts with Python | Manual creation using the graphical user interface |
Reduced technical debt | Helps organizations avoid building up technical debt in their data processes | Workflows often become complex and difficult to maintain over time |
Database connections and APIs | Yes, both native integrations with databases and the ability to set up API requests | Through PowerQuery |
Learn why people are using Quadratic as a better alternative to Excel.
3. Python (pandas): Programmer's choice for data cleaning
For those comfortable with coding, Python's Pandas library offers unparalleled flexibility for data cleaning in Python:
- Powerful data structures: DataFrame objects designed specifically for data manipulation
- Vectorized operations: Perform operations on entire columns efficiently
- Advanced filtering: Complex conditional logic for identifying data issues
- Regular expressions: Pattern matching for text cleaning
- Integration with visualization tools: Easy transition to analysis after cleaning
Python for data cleaning has become increasingly popular as more organizations adopt programming-based approaches to data preparation. The extensive availability of Python libraries makes cleaning data with Python flexible and scalable.
4. OpenRefine: The specialist's tool
Formerly Google Refine, OpenRefine offers specialized functionality for messy datasets:
- Clustering algorithms: Identify similar entries that might represent the same value
- Text faceting: Explore and clean text data efficiently
- GREL expressions: A specialized language for data transformations
- Web scraping capabilities: Extract and clean data from HTML
- Reconciliation services: Match local data against external databases
OpenRefine excels at discovering patterns in messy data and handling large text-based datasets where inconsistencies are common.
5. Alteryx Designer Cloud (previously Trifacta): Enterprise-grade cleaning
For organizations dealing with massive datasets and complex data pipelines, Alteryx Designer Cloud offers industrial-strength capabilities:
- Visual data profiling: Automatically identifies anomalies and patterns
- Intelligent sampling: Efficiently works with large datasets
- Predictive transformation: Suggests cleaning operations based on data structure
- Collaboration features: Enables team-based cleaning workflows
- Enterprise security: Maintains data governance during cleaning processes
The platform emphasizes data quality and governance, making it suitable for regulated industries where data integrity is paramount.
Best practices for data cleaning
Regardless of which tools for cleaning data you choose, these best practices will improve your outcomes:
- Document your cleaning process: Create a record of transformations applied to raw data.
- Start with a copy: Never modify original datasets directly.
- Look for patterns in errors: Systematic issues often have systematic solutions.
- Validate results: Verify that cleaning hasn't introduced new problems.
- Automate repeatable processes: Build templates or scripts for recurring cleaning tasks.
Data cleaning is evolving with AI
The future of data preparation lies in AI-assisted approaches. AI for data cleaning is revolutionizing how we handle data quality issues:
- Anomaly detection: Automatic identification of outliers and unusual patterns
- Smart imputation: Suggesting appropriate values for missing data
- Format standardization: Automatically normalizes inconsistent formats
- Entity resolution: Identifies when different records refer to the same entity
- Continuous learning: Improves cleaning suggestions based on user feedback
Platforms like Quadratic represent the cutting edge of this trend, where AI data cleaning capabilities are built directly into the analysis environment.
Conclusion
It can be difficult to choose the right tool for your needs. Data cleaning represents a critical but often underappreciated step in the analytics process. The best data cleaning tools balance data automation with control, giving you confidence in your data's integrity without excessive manual effort.
For organizations looking to minimize or eliminate the cleaning phase entirely, Quadratic's AI-powered approach offers a compelling vision of the future. For those deeply invested in programming workflows, Python with Pandas provides unmatched flexibility. Excel remains accessible for widespread adoption, while OpenRefine and Alteryx Designer Cloud serve specialist needs and enterprise requirements, respectively.
Ultimately, the right choice depends on your team's skills, dataset complexity, and integration requirements. By investing in appropriate data cleansing tools, you can transform the most tedious part of analysis into a streamlined process, allowing your team to focus on extracting valuable last-mile insights rather than wrestling with messy data.