MJ Lindeman, PhD, Community Partner
Apr 1, 2025
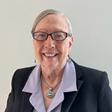
In a data-driven competitive business environment, the ability to work effectively with data has become a fundamental skill across virtually every industry. However, an important distinction exists between merely understanding data and truly mastering it. This distinction is captured in the concepts of data literacy vs data fluency. These two related but distinct competencies are increasingly recognized by organizations as essential for competitive advantage.
The emphasis on data skills is no longer limited to just a few specialized departments in a company. In 2022, Forrester Consulting surveyed experienced employees and decision-makers at global companies with more than 500 employees. For employees across the 10 departments listed in the following table, 80% to 92% of the respondents defined basic data skills as important or very important for their work.
Department | Percentage |
---|---|
Customer insights | 89% |
Analytics | 85% |
Finance/Accounting | 80% |
HR/Training | 86% |
IT | 90% |
Marketing/Advertising | 87% |
Operations | 86% |
Product | 92% |
Research | 83% |
Engineering | 81% |
To emphasize the importance of these numbers, consider that data skills were ranked higher than traditional skills such as project management skills, computer skills, and communication skills.
The difference between data literacy vs data fluency
Data literacy represents the foundational ability to read, understand, explore, use, and communicate using data in context. It's about having the basic skills to interpret data visualizations, understand simple statistics, and draw basic conclusions from information presented in charts or tables. A data-literate individual can answer questions like "What happened?" or "How many?" but might struggle with more complex analytical queries.
In contrast, data fluency represents a more advanced relationship with data. Data-fluent individuals don't just understand data. They can manipulate it, transform it, and leverage it to generate genuine insights. They can formulate meaningful questions, determine appropriate methodologies, and communicate nuanced findings with confidence. Data fluency skills enable people to address deeper questions like "Why did this happen?" and "What actions should we take?"
To illustrate the difference with a practical example: a data-literate marketing manager might be able to read a dashboard showing campaign performance metrics and identify which campaigns had the highest click-through rates. Data-fluent managers, however, could dig deeper. They might connect that performance data with customer segments, identify meaningful patterns in user behavior, and recommend specific optimizations backed by quantitative evidence.
The gap between these competencies represents a significant challenge for organizations pursuing data-driven transformation. While many employees achieve basic data literacy through training programs, the transition to true fluency often remains elusive, creating a barrier to extracting maximum value from data investments.
The organizational impact of the data skills gap
In many organizations, this skills gap manifests as a bottleneck in the analytical workflow. Data-literate employees identify opportunities in data but lack the technical skills to pursue them independently. They become dependent on specialized teams, creating delays and frustration.
This gap also impacts how effectively teams collaborate around data. In meetings, data-literate employees might acknowledge the importance of metrics without feeling empowered to challenge assumptions or suggest alternative analytical approaches. The result is a passive relationship with data rather than an active dialogue. This is precisely the opposite of the data-driven culture most organizations are striving to build.
The traditional approach to bridging this gap has focused on more training. This typically means teaching employees SQL, Python, or statistical methods. While valuable, this approach faces practical limitations. Most employees lack the time for extensive technical education alongside their primary responsibilities. The solution isn't necessarily more training but better tools that meet users where they are while elevating their capabilities.
How AI is changing the data competency landscape
The emergence of AI business intelligence tools is fundamentally reshaping how we think about the progression from data literacy to data fluency. Rather than forcing users to climb a steep technical learning curve, these tools provide scaffolding that supports more sophisticated interaction with data.
Consider how AI transforms the query process itself. Instead of requiring formal query languages, AI interfaces accept natural language questions. A marketing manager can ask, "Which customer segments showed the biggest response to our holiday campaign?" rather than struggling to construct a complex SQL query joining multiple tables. This natural language interface removes a significant technical barrier while preserving the analytical depth that characterizes true data fluency.
AI also accelerates the interpretation process. Beyond generating visualizations, modern AI systems can highlight anomalies, identify potential correlations, and suggest possible causal factors. These capabilities help bridge the gap between seeing data and understanding its implications, taking AI-powered data literacy a critical step toward fluency.
Perhaps most importantly, AI systems can guide users toward better analytical practices. By suggesting relevant variables, recommending appropriate visualization types, or flagging potential confounding factors, AI becomes both a tool and a teacher, gradually building users' analytical intuition through practical application rather than abstract training.
Quadratic fosters data literacy and data fluency
Quadratic's approach to data analysis represents a particularly effective model for bridging the gap between basic data literacy and comprehensive data fluency. By combining the familiar spreadsheet paradigm with AI-powered capabilities and code flexibility, Quadratic creates an environment where users can progressively develop their data skills while being productive.
For data-literate users, Quadratic provides an accessible entry point through its spreadsheet interface. Users can upload their data, create simple visualizations, and perform basic calculations using familiar formulas. The spreadsheet paradigm leverages existing skills, minimizing the initial learning curve and allowing users to build confidence through early successes.
As users grow more comfortable, Quadratic's AI capabilities help them progress toward greater fluency. The ability to ask natural language questions about data represents a crucial transitional capability. A user who might not know how to write a complex query can still ask sophisticated questions and receive immediate feedback, gradually developing a more nuanced understanding of data relationships.
For example, a sales manager reviewing quarterly performance might start by looking at a simple summary of results. Using Quadratic's AI interface, they could then ask, "What factors most strongly correlate with our high-performing regions?" The system would generate the appropriate analysis, showing the correlation between sales performance and various potential factors like team size, marketing spend, or customer demographics.
This interactive Q&A process accomplishes two objectives simultaneously: it delivers immediate analytical value while demonstrating analytical techniques the user might later adopt independently. The user doesn't just get an answer. They see how that answer was derived, establishing a model for future exploration.
For users ready to take the next step toward fluency, Quadratic offers native support for Python, SQL, and JavaScript. The AI can generate starter code for common analytical tasks, allowing users to begin working with code without starting from scratch. They can modify and extend these code snippets, gradually taking greater control of the analytical process while still having the safety net of AI assistance.
The path to organizational data fluency
Organizations seeking to foster greater data fluency can leverage AI-powered tools like Quadratic as part of a comprehensive strategy. Here's how such a strategy might unfold:
- Start with access and transparency: Ensure that data is accessible to employees across the organization, with appropriate governance controls. Quadratic's direct database connections help break down data silos while maintaining security and compliance.
- Encourage exploration: Create a data culture and literacy environment where asking questions of data is encouraged. Quadratic's AI-powered interface removes the technical barriers to data exploration, allowing employees to pursue their curiosity without specialist intervention.
- Provide scaffolding, not just training: Rather than requiring extensive upfront training, provide tools that offer progressive assistance. Quadratic's combination of AI guidance and code generation allows users to gradually increase their technical involvement as their skills develop.
- Facilitate collaboration between technical and non-technical users: Tools like Quadratic enable mixed-skill teams to collaborate effectively, with each member contributing according to their capabilities. Technical users can create sophisticated analyses that non-technical users can then explore and extend through the AI interface.
- Recognize and reward data-driven decision-making: Create incentives for employees to incorporate data into their decision processes, regardless of their technical skill level. Quadratic's ability to generate clear visualizations and insights helps employees at all levels present data-backed recommendations.
The future of data skills development
There has been a focused effort to teach data literacy in schools, and now the focus is moving to data fluency. Data skills are becoming required for all workers, and the expected level of data skills is increasing. A 2022 Forrester survey found that company decision-makers and employees believe basic data skills are "the most important skills for their employees to succeed in their day-to-day work." Decision-makers expect advanced data skills to increase most in importance.
Looking ahead, the boundary between data fluency vs data literacy is likely to become increasingly fluid. AI-powered data literacy tools will continue to democratize access to sophisticated analytical techniques, allowing more employees to perform analyses that once required specialized training.
This doesn't mean that technical skills will become irrelevant. Rather, the technical threshold for meaningful data work will shift. Understanding the principles of sound analysis will remain critical. Concepts like causation versus correlation, selection bias, or statistical significance will still matter. However, implementation details will increasingly be handled by AI systems, allowing humans to focus on the creative and strategic aspects of data work.
Organizations that embrace this hybrid approach, combining human judgment with AI-powered analysis, will gain significant advantages. Their employees will progress more quickly from basic literacy to genuine fluency, accelerating the organization's overall self-serve analytical capabilities and fostering a truly data-driven culture.
By providing tools like Quadratic that meet users where they are while elevating their capabilities, organizations can bridge the gap between data literacy vs data fluency. The result is a workforce empowered not just to read data but to speak its language fluently, generating the meaningful insights that drive innovation and competitive advantage in today's data-rich business environment.