MJ Lindeman, PhD, Community Partner
Jan 28, 2025
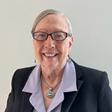
In today's data-driven world, organizations invest heavily in sophisticated analytics tools and platforms. Yet many still struggle with what is called "last-mile analytics,” which is the final step where insights are recognized and transformed into action.
But what exactly is an insight in this context? Far more than a data point or a simple observation, a true insight in the last mile of analytics represents a bridge between understanding and action. As this article describes, “the challenge is not only understanding AI but leveraging it effectively to unlock efficiency, drive innovation, and stay ahead of the competition. “
Data, information, and insights
Data, information, and insights are very different things. Data can be a sequence of numbers, such as 6148997776. It becomes information when you recognize it as a 10-digit telephone number, and it becomes knowledge when you know the name associated with that number. Data, information, and knowledge are necessary for an insight to happen, but having an insight requires an additional step in your thinking.
Consider the difference between information, knowledge, and insight through a practical example. When an analytics dashboard shows that website traffic increased 25% last month, that's information. When analysis reveals this increase coincided with a new campaign launch, a knowledge pattern has been identified.
A genuine insight comes by realizing the traffic surge came primarily from mobile users searching specific terms. This reveals both an opportunity and a clear path to action, such as optimizing mobile landing pages to serve these users. An actionable insight connects (a) knowledge gained from an analysis to (b) knowledge of an action that will produce value.
This transformation from data to actionable insight lies at the heart of last-mile analytics and quality. As organizations advance their last-mile data analytics capabilities, they are discovering that truly valuable insights share several crucial characteristics. First, they provide a contextual understanding that connects data patterns to specific business situations. This reveals not just what is happening, but why.
Secondly, actionable insights carry clear business implications that identify specific opportunities or risks and their potential outcomes. Perhaps most importantly, they arrive at the right moment, when decisions can still be made and actions can be taken.
The last mile in data analysis is where these insights must find their way into decision-makers' hands in a form they can act upon. Modern end-user analytics have made information and knowledge more accessible than ever so that more insights can occur.
However, the challenge remains in ensuring valuable insights reach their destination: the decision-maker's mind. Just as delivery services face their biggest challenges in the final mile of package delivery, organizations face similar hurdles in ensuring analytics insights drive real business value.
Why the last mile of analytics is so difficult
The concept of last-mile analytics and quality extends far beyond creating dashboards or running reports. As organizations mature their last-mile data analytics capabilities, they're discovering that success demands a fundamental shift in how we think about data delivery. Despite access to powerful self-serve analytics tools, many organizations remain insight-rich but action-poor, struggling to bridge the gap between knowing and doing.
According to industry research, while most large enterprises have robust data infrastructure, many struggle to extract meaningful value from their investments. Organizations that master the last mile see significantly higher returns on their analytics investments. Unfortunately, the more severe challenge to mastery is from people, not technology.
The journey of transforming raw data into actionable insights faces several significant challenges that make the last mile of analytics particularly demanding. At its core, last-mile analysis involves bridging the gap between technical capabilities and human understanding. This challenge manifests in multiple ways.
Perhaps the most pervasive obstacle is cognitive overload. End-user analytics tools have become increasingly sophisticated, but this sophistication often comes at the cost of complexity. When users face an overwhelming amount of information without clear prioritization, they can become paralyzed by competing data narratives. This analysis paralysis prevents effective decision-making, defeating the very purpose of analytics implementation.
Cultural resistance presents another formidable barrier in last-mile analytics and quality implementation. Many organizations find that entrenched decision-making habits are difficult to change, even with better data available. There's often an underlying fear of measurement and accountability that comes with data-driven transformation and decision-making. This resistance is particularly challenging when implementing self-serve analytics initiatives, as users may prefer familiar, if less effective, ways of working.
Technical friction adds another layer of difficulty. Complex access procedures, inconsistent experiences across platforms, and poor performance can frustrate users and discourage adoption. When the last mile in data analysis becomes a technical obstacle course, even the most valuable insights may never reach their intended audience. This article in the Harvard Business Review provides an example.
Building a bridge across the last mile
Success in the last mile requires a thoughtful approach to three fundamental areas: insight translation, engagement design, and action catalysis.
- Insight translation is the process of distilling complex data patterns into contextual business recommendations that non-technical decision-makers can confidently act upon.
- Engagement design involves thoughtfully structuring analytics interfaces and workflows to match how different users naturally approach problem-solving and decision-making.
- Action catalysis is the systematic connection of data insights to specific business opportunities, creating additional motivation and momentum that produces organizational change.
The key to effective insight translation lies in transforming complex findings into compelling stories that resonate with decision-makers. This means going beyond simple data visualization to create narratives that connect data patterns to business opportunities. There are times it requires digging deeply to understand what the organization needs to gain or lose so it can move forward in a specific direction.
Engagement design focuses on building intuitive pathways to discovery. This involves understanding how different users interact with data and creating experiences that match their decision-making styles. End-user analytics tools must be designed with the user's journey in mind, creating moments of discovery that build confidence and encourage deeper exploration.
An insight is a catalyst that quickens actions. Action catalysis is perhaps the most crucial element, and it may be the most difficult to achieve. Understanding insights is not enough; they must trigger behavior change. This requires establishing clear connections between insights and actions, creating accountability for data-driven decisions, and building feedback loops that demonstrate the value of decisions.
Creating momentum for change
Successful last-mile analytics implementations start with small wins and build momentum through visible successes. By focusing first on motivated users and high-value decisions, organizations can create success stories that inspire broader adoption. This approach helps overcome initial resistance and demonstrates the practical value of data-driven decision-making.
The key is to optimize for impact rather than aim for perfection. By removing unnecessary complexity and creating clear feedback mechanisms, organizations can help users build confidence in their ability to use data effectively. This confidence is crucial for the successful adoption of self-serve analytics platforms and the development of a data-driven culture that yields actionable insights.
Measuring progress and success
The journey to effective last-mile analytics requires a comprehensive measurement framework that captures both immediate impact and long-term value creation. The most successful organizations track three distinct categories of metrics to evaluate their progress.
- Adoption (initial usage) metrics reveal how well the organization is embracing data-driven decision-making. These include not just basic usage statistics of end-user analytics tools, but deeper measures like active user engagement patterns, time spent reaching insights, and most importantly, the frequency of collaborative data discussions in decision-making meetings. User confidence scores and self-reported comfort with analytics tools provide valuable feedback about adoption barriers and training needs.
- Value (perceived worth) metrics demonstrate the tangible impact of last-mile analytics initiatives. Decision speed improvement is a crucial indicator. How much faster can teams move from data to action? Revenue impact and cost avoidance provide clear financial justification for analytics investments. Quality metrics track the accuracy and reliability of insights, while utilization rates show which analytics capabilities deliver the most value.
- Transformation (long-term impact) metrics measure the organization's progress toward becoming truly data-driven. These include tracking how analytics maturity evolves across different departments, the sophistication of questions being asked of the data, and the increasing complexity of decisions being supported by analytics. Cultural indicators like the number of employees actively championing data-driven approaches and the integration of analytics into standard operating procedures help gauge the depth of transformation.
Beyond these categories, organizations should also monitor the health of their self-serve analytics implementation through metrics like support-ticket resolution times, user satisfaction scores, and the ratio of self-service to analyst-dependent requests. These operational measures help identify friction points in the last mile of analytics delivery.
As analytics technology evolves, new opportunities are transforming how organizations approach the last-mile challenge.
- Predictive insight delivery uses AI to proactively identify and surface relevant insights to users before they need to ask for them.
- Context-aware analytics automatically adapts how information is presented based on the user's role, situation, and current business context.
- Decision automation creates direct connections between insights and actions by implementing routine decisions automatically based on predefined rules and learned patterns.
These advances, combined with improvements in user experience design and data visualization, are making it easier to bridge the gap between an insight and deploying it as an action.
Looking ahead
The future of last-mile analytics lies in creating more intuitive, more integrated experiences that seamlessly connect insights to actions. As organizations continue to invest in end-user analytics tools and capabilities, the focus must remain on the human element of data-driven decision-making. Success requires not just technical excellence in last-mile data analytics, but also a deep understanding of how people interact with data and make decisions. The organizations that thrive are those that master both the technical aspects of self-serve analytics and the human elements of insight delivery.
Remember, the goal of last-mile analytics and ad-hoc reporting is not just to analyze data. It is to create positive change through better, data-driven decisions. By mastering the last mile in data analysis, organizations can transform their analytical capabilities into tangible business outcomes. While the journey may be challenging, the rewards of successful last-mile analytics delivery make it worth the effort.
Organizations that succeed in this transformation will be those that know the core issue is a people issue. By focusing on this crucial final step and addressing both the technical and human elements, investments in data analytics modernization can truly pay off by creating lasting value and a competitive advantage.